Adaptive AI/ML for Dynamic Cybersecurity Systems
The application of AI/ML to cybersecurity (AI4SEC) is increasingly becoming a paramount need to protect our digital societies from cybercrime. In practice, AI4SEC faces many challenges which need rapid answer. DynAISEC advances the AI4SEC domain in three critical aspects: it
- improves the availability of big data for AI/ML model training through automatic data synthetization and augmentation techniques
- makes AI4SEC robust to the dynamic nature of cybercrime, by integrating adaptive and incremental learning in AI4SEC models, and
- improves AI4SEC transparency, trust, and usability through the adoption of explainable AI technology. DynAISEC aims at empowering an Austrian and European initiative in AI4SEC and cybersecurity data independence.
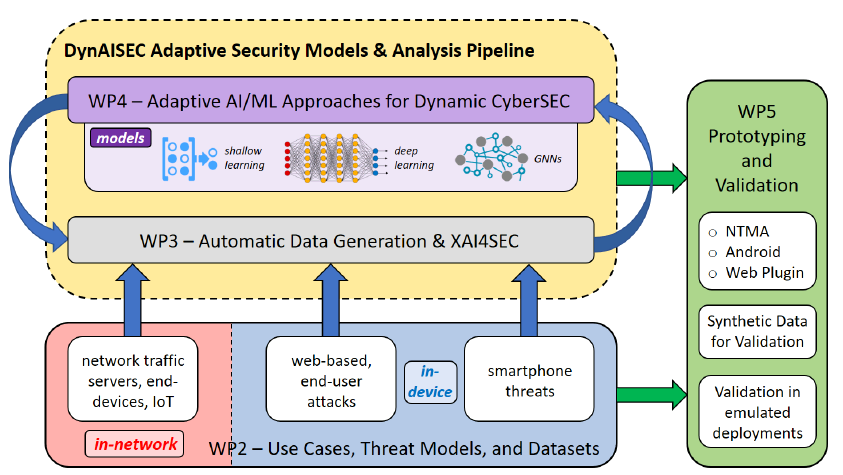
Motivation
Cybersecurity is a cornerstone to our digital society. The rise of ubiquitous mobile communications, the plethora of heterogeneous interconnected devices (IoT, smartphones, M2M, etc.), the growing number of critical infrastructures served over networks (e-health, finances, governance, etc.), and the massive shift of society to an all-remote paradigm induced by the COVID-19 pandemic have nothing but accelerated the pace of new cyber threats and the realization of cyber-attacks impacting all sorts of systems and enterprises, calling for better, more efficient, and more robust approaches to cybersecurity. The impressive success of Artificial Intelligence (AI) and Machine Learning (ML) in multiple data-driven problems over the past decade has motivated a flourishing research domain targeting the application of AI/ML to cybersecurity problems – AI4SEC. However, making of AI4SEC an accepted and fruitful approach to cyber security in the practice has proven extremely challenging.
Goals
The goal of DynAISEC is to significantly advance the AI4SEC domain, tackling one particularly complex deficiency faced by currently proposed solutions, which limits their application in practice: the lack of adaptive and incremental learning to deal with the dynamic nature of cyber-attacks and networking data. Most AI4SEC models are trained offline, causing them to underperform in the event of previously unseen attacks (high rates of false negatives) or under dynamic baseline scenarios (high false alarm rates). The ability to retrain AI4SEC models as new data is generated is fundamental to achieve adaptive incremental learning, but remains an open research direction. To tackle this limitation, we propose to research and develop the GenDeX technology: GenDeX stands for a combined approach to adaptive cybersecurity, spanning three key components:
- the automatic and continuous Generation of synthetic cybersecurity data through generative models – synthetizing and augmenting input data in controlled yet heterogeneous scenarios to enhance data-driven learning
- the continuous Detection of attacks and anomalies through novel AI4SEC models – from Deep to Graph-based learning architectures – exploiting recent advancements in AI/ML, as well as the Detection of so-called concept-drifts – changes in the underlying statistics of the analyzed data (either attacks or baseline), to decide for the right times to (incrementally) re-train the conceived AI4SEC models; and
- the automatic eXplanation of both AI4SEC detection decisions as well as concept drift detections, to further increase understanding and trust on AI4SEC, additionally enabling faster means for diagnosis and decision making.
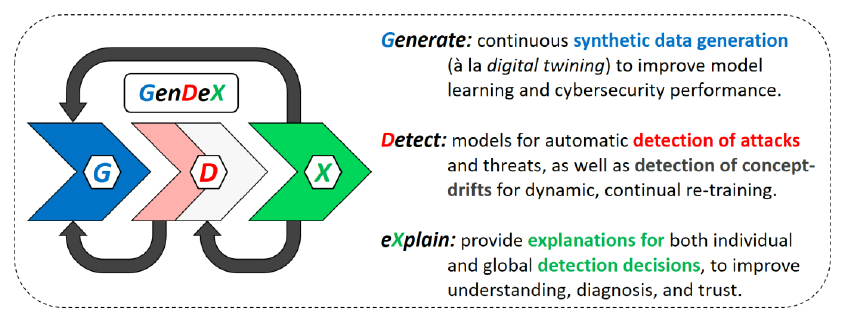
Expected findings
The expected results of DynAISEC are:
(i) data-driven AI4SEC models with adaptive capabilities for enhanced cybersecurity performance (higher detection rates with lower false alarms)
(ii) algorithms for the automatic synthetization and augmentation of cybersecurity data for adaptive model re-training, offering data curation warranties – i.e., the correctness of the synthesized data
(iii) software libraries providing explainability of model behaviors and predictions
(iv) multiple prototype demonstrators targeting three specific cybersecurity use cases, including in-network security (malware propagation, IoT targeted attacks. DDoS attacks), web-browsing end user security (phishing attacks, fake site detection, privacy leakage, employee data exposure), and in-device security for mobile devices (malware detection in smartphones).
Further Information
- The project is led by the University of Vienna, the official project lead is Johanna Ullrich.
- SBA Research is a project partner.
- Project website
Contact
This project is funded by the FFG.